In his quest to develop an AI capable of understanding many different dialects, Meta created an AI model, SeamlessM4T, which can translate and transcribe nearly 100 languages from text to speech. Open source with SeamlessAlign, a new translation dataset, Meta says that SeamlessM4T represents a "significant advance" in speech-to-speech and speech-to-text. "Our unique model provides on-demand translation that allows people who speak different languages to communicate effectively," Meta writes in a blog post shared by TechCrunch. "SeamlessM4T fully recognizes the source language without the need for a separate language recognition system."SeamlessM4T is the successor to Meta's No Language Left Behind, a type of text-to-text machine translation, and Universal Speech Translator, one of the few speech translation systems to support the Hokkien language. Powered by Massively Multilingual Speech, the Meta system provides speech recognition, language recognition, and text-to-speech technology in over 1,100 languages. Meta is not alone in investing in creating sophisticated AI rendering and texting tools. In addition to the wealth of commercial services and open models currently available from Amazon, Microsoft, OpenAI, and many startups, Google is developing what it calls the Universal Voice Model, as part of an effort by the tech giant to demand creating a model that can. of understanding 1,000 of the world's most spoken languages. Mozilla, meanwhile, has launched Common Voice, one of the largest collections of multilingual voices for training automatic speech recognition algorithms. But SeamlessM4T is one of the strongest efforts to date to combine translation and transcription capabilities into a single model.
In its creation, Meta claims to have released publicly available text (in the order of "ten billion" sentences) and speech (4 million hours) on the web. In an interview with TechCrunch, Juan Pino, a research scientist in Meta's AI research department and a contributor to the project, did not reveal the exact source of the data, saying only that there were "different types."Not all content creators agree with the practice of mining public data to train marketable brands. Some have filed lawsuits against companies that build AI tools from publicly available data, arguing that vendors should be required to offer credits, even refunds, and clear opt-outs.But Meta says the data it releases — which may contain personally identifiable information, the company admits — is not copyrighted and comes from open source or licensed sources. However, Meta used the retrieved text and speech to create a training dataset for SeamlessM4T, called SeamlessAlig. Researchers combined 443,000 hours of speech and text and created 29,000 hours of "speech", which "taught" SeamlessM4T how to transcribe speech and text, translate text, create speech from text, and even translate these words into - speak the same language. into words in another language. Meta says that, according to internal benchmark tests, SeamlessM4T performed better against background noise and "speaker distortion" in text-to-speech operations compared to current state-of-the-art voice-to-speech models. It says this is a rich combination of speech and text in the training dataset, which Meta says gives SeamlessM4T an edge over speech and text alone.
"With positive results, we believe that SeamlessM4T is a big step forward in AI's quest to create a universal multitasking system," Meta wrote in a blog post. But one can only wonder what kind of thinking this type can have. A recent article from The Conversation highlights several flaws in AI-based translation, including various forms of gender bias. For example, Google Translate once assumed that doctors were male while nurses were female in some languages, while Bing Translator translated words like "sweet table" as the female "die Tabelle" in German, which refers to different numbers. Algorithms for speech recognition are also biased. A study published in The Proceedings of the National Academy of Sciences found that a voice recognition system from a large company was twice as likely to incorrectly transcribe the voices of black speakers as compared to those of non-whites. white tongue.
Unsurprisingly, SeamlessM4T is not unique in this regard. In a white paper published on the side of the blog, Meta reveals that the model "extends to the masculine form when translating from the neuter word" and works well when translating from masculine references (for example, nouns like "he" in English) for many languages. In addition, in the absence of gender information, SeamlessM4T chooses to translate the gender about 10% of the time - possibly due to the "overrepresentation of male words" in the training data, Meta speculates. Meta argues that SeamlessM4T doesn't include a fatal text limit in its translation, a common problem with translation and AI-generated text types in general. But it is not enough. In some languages, such as Bengali and Kyrgyz, SeamlessM4T makes more lethal translations - i.e. hateful or meaningless translations - about socio-economic and cultural contexts. And in general, SeamlessM4T is a killer in the translation that talks about the process of sex and religion. Meta says that SeamlessM4T's public display has filters for toxic word submissions as well as filters for potentially toxic words. However, this filter is not available by default in open-source versions. A big problem with non-verbal AI translators in the white paper is the loss of verbosity that can result from overusing them. Unlike AI, human translators make their own choices when translating from one language to another. They can be interpreted, corrected, or collected and aggregated, creating a fingerprint known informally as a "translation." AI systems can produce more "accurate" translations, but these translations may be missing translations in different contexts. This is probably why Meta advises against using SeamlessM4T for long translations and colorized translations, as recognized by government agencies and translation leaders. Meta also discourages the deployment of SeamlessM4T for medical or legal reasons - perhaps an attempt to cover its base if there is no translation. It is wise; there have been at least a few times where errors in AI translation have led to mistakes in law enforcement. In September 2012, police charged a Kurdish man with supporting terrorism over redacted text messages. In 2017, a Kansas cop used Google Translate to ask a Spanish speaker if he could search his car for drugs, but because the translation wasn't accurate, the driver didn't quite understand what he had. later lost.
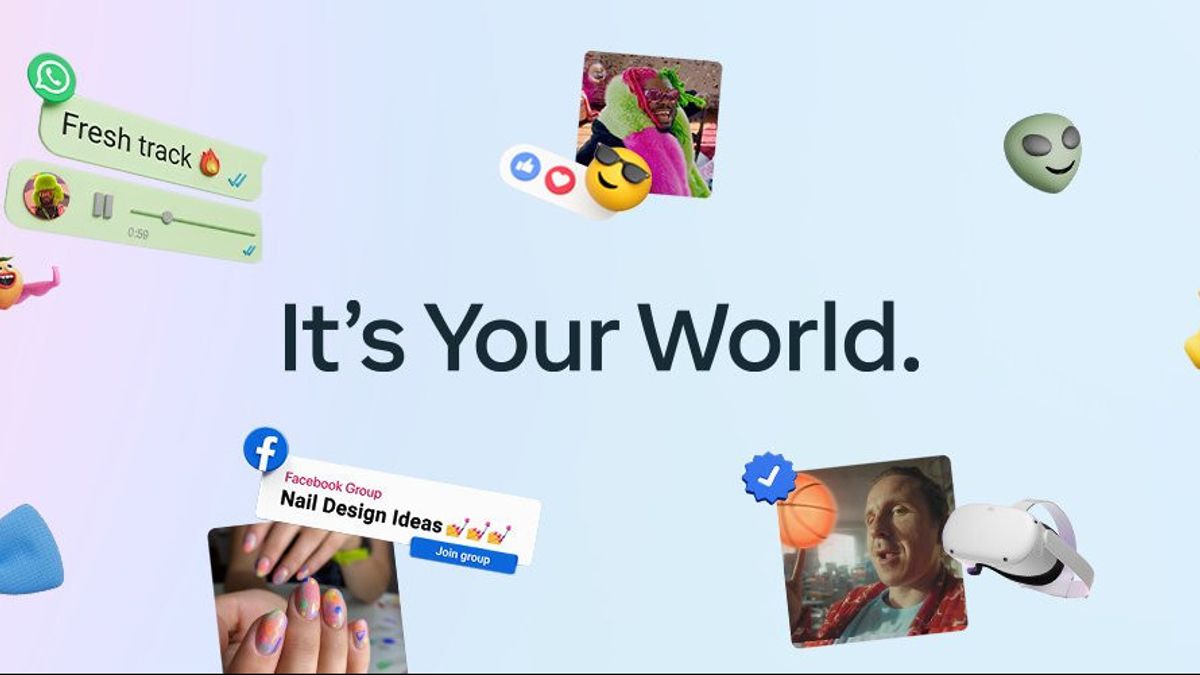
“Our single model provides on-demand translations that enable people who speak different languages to communicate more effectively,” Meta writes in a blog post shared with TechCrunch. “SeamlessM4T implicitly recognizes the source languages without the need for a separate language identification model.” “With state-of-the-art results, we believe SeamlessM4T is an important breakthrough in the AI community’s quest toward creating universal multitask systems,” Meta wrote in the blog post.
“This single system approach reduces errors and delays, increasing the efficiency and quality of the translation process, bringing us closer to making seamless translation possible,” Pino said. “In the future, we want to explore how this foundational model can enable new communication capabilities — ultimately bringing us closer to a world where everyone can be understood.”